The Power of Small Data: When stymied, make your own database
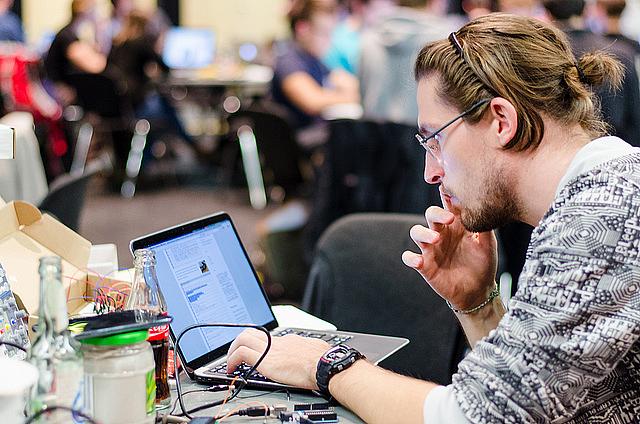
You went hunting for data for your next award-winning health story but came up skunked.
You asked for data. But the agency told you it didn’t have any data. And you could not prove them wrong. Do you give up?
No. You grow your own.
From dangerous doctors to dangerous candy to dangerous dirt, I have been involved in project after project that started with little more than an empty spreadsheet and the will to fill it.
That will must endure, too. Because building a database can be extremely frustrating. It requires less technical know-how than you might think and lots of patience.
Take up yoga if you need to. Have your partner shaking the cocktails by the time you get home at whatever hour. Anything you need to do to keep pushing. The closest thing I have experienced to it was climbing Mount St. Helens. The final stretch is just volcanic ash. If it’s a hot day – which it was – that means you are already tired and sweating and now every step you take seems to send you farther down the mountain.
The way you end up slipping back down the mountain of data most often is by not thinking through all the different categories of data you would like to create. For example, you might type in the names of physicians who have been disciplined by your state medical board. You put in the last name in one field, the first name in another, their work address, their license number, and the type of discipline they received. This takes you the better part of two weeks.
Then later you decide that you are interested in whether many of these physicians happen to be older. (True story.) So you have to go back and create a field just for the date that the person earned a medical license (a proxy for age, but still). Then you have to go back through all your paper documents and type in the license date.
To save yourself from repetitive stress injury, it’s even better if you can stitch together a bunch of datasets that someone else has created.
I ended up doing a little bit of the build-your-own method and the stitch-it-together method most recently when I was working with a team of reporters on the Just One Breath series about the lack of attention being paid to valley fever. Amazingly, the state of California told us that they had no digital data to share with us. They had stacks of paper files. But they said that it would be cost- and time-prohibitive to scan and send them all to us.
So we went to the counties that had known valley fever cases. We asked how they tracked valley fever cases and, after calling and calling and emailing and emailing, we ended up with a nice little set of digital records. A data hodgepodge, for sure, but useful nonetheless.
I pulled everything together into one spreadsheet and found out quickly that things didn’t line up. They rarely do. At this point, I would normally call a data expert. I wrote about how to do that in a previous post. They can help you “crosswalk” between datasets. Because I have worked with so many data experts over the years, I felt I had a good enough grounding to link the data together on my own. This usually means finding items in one dataset that are either exactly like — or can be easily adapted to be like — the items in another dataset. For example, if both datasets include county names, you can set them both up so the county names are in the same column. That way when you bring the datasets together, they line up. You will have whole columns where there is no information because one of your datasets did not contain that information. But if you have the critical information, such as the number of valley fever cases reported by year in each county, then you can start running some calculations.
Your next question should be, How do I use a spreadsheet that I have built from scratch to find a story? Joshua Hatch wrote a great spreadsheet “how to” for Poynter that you can find here.
Next: How to handle data haters.
[Photo by TechCrunch via Flickr.]