How I kept the battle for data from slowing down my story on racial bullying
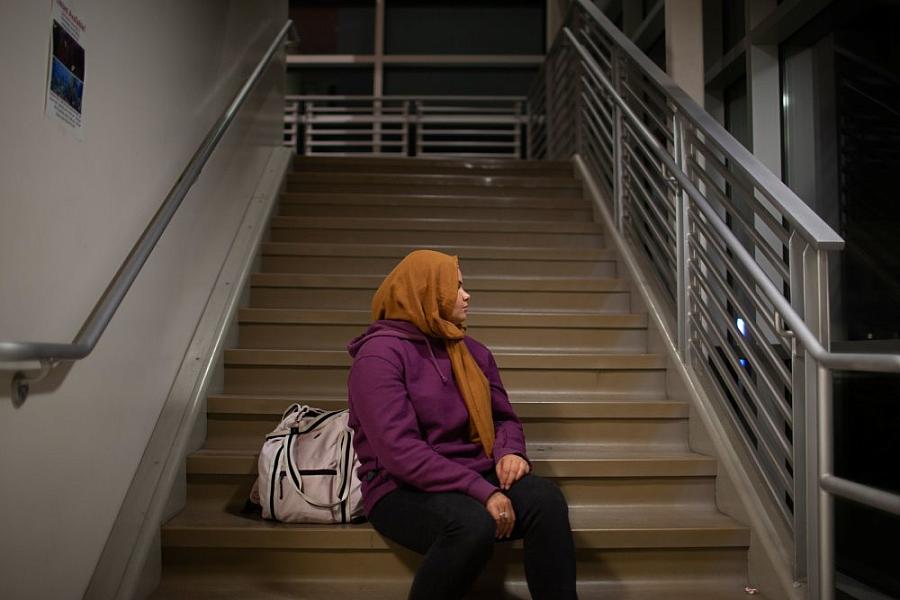
Aneesah's teacher directed discriminatory comments at her, and ripped the hijab off her head. (Photo: Kevin German/Luceo for USN&WR)
A small study published in late 2018 found that “adolescents’ behavioral responses to recent societal expressions of discrimination may warrant public health attention” and policy intervention. That claim caught my attention, and I wanted to advance and elevate it. I knew there was data to do so, but I had no idea how hard it would be to get. Preparation and persistence proved key.
The initial study of about 2,600 adolescents in Southern California found that those who reported concern about discrimination were more likely to use substances such as cigarettes, alcohol and marijuana. Similar questions about cigarette, alcohol, marijuana and other types of drug use, as well as experiences of bias-related bullying, are asked of more than 300,000 public high school students each year in the California Healthy Kids Survey. With that survey data from the California Department of Education, I had a sample size that was more than 100 times larger than the initial study and was able to expand the scope of the analysis statewide.
I reported from my own analysis of the 2017-18 school year data that students in California public high schools who said they’d been bullied because of their race, ethnicity, or national origin were twice as likely to have smoked cigarettes. Alcohol consumption also was higher – 40% among students who had suffered this bias-related bullying, compared with 29% among those who had not — as were reported usage rates for marijuana, cocaine and heroin, and for prescription opioids, sedatives or tranquilizers.
The public records request process with the California Department of Education was a true battle. It took nearly six months to receive data files with anonymized student responses to the survey for the five most recent school years, despite a state law that allows for just 10 business days for a response. With deadlines looming, I was able to publish a feature-length story just a week after receiving data files with tens of millions of data points. I wouldn’t have been able to do that without a very clear understanding of the scope and limitations of the data set I used from very early on.
Here are three times when it proved critical to have a very focused question that I aimed to answer with the data:
1) Getting the data
Because I knew the question I wanted to use the data to answer, I was able to respond quickly when the California Department of Education tried to compromise with me on my public records request. They argued that I couldn’t receive individual-level student responses because I wasn’t a “researcher.” But I knew: 1) thanks to my mentor, Paul Overberg, that responses to public records requests can’t discriminate based on job title and 2) that I couldn’t compromise on this point. Anonymizing student names would be fine (and expected), but I needed to connect the risky health behaviors of a student to their individual experiences with bias-related bullying. Data summarized more broadly than the individual level wouldn’t work for that. I preferred school district identifiers for the student responses, but settled on county identifiers, as that specification wasn’t as critical to the point I planned to make in this piece. Also, being able to explain to my team how getting the answer to this question would make the story stronger helped earned their trust and support, keeping them on board through the many setbacks over the full six months.
2) Reporting while waiting for data
As the California Department of Education continued to drag their feet in responding to my public records request, I quickly realized I would have to do much of my reporting before I received the data. Early interviews aligned with the findings from the study that initially sparked my interest in the topic, so I knew I was on the right track. I had a hypothesis — that teens who experience bias-related bullying were more likely to smoke or drink — and that hypothesis served as a general nut graf until I was able to fill in the specifics of exactly how much more likely with the data. I ran with that hypothesis — posing that generalized nut graf to lawmakers, activists and students — which helped me to have about 80 percent of my story written and through an initial edit before the data was delivered.
3) Running the analysis
Perhaps most importantly, when I finally did receive the data, I was prepared to create a pivot table in Excel that would answer my main reporting question. Research on the state agency’s website gave me a general sense of what the data file would look like, so I was able to plan which fields would go where in the pivot table building tool. It took my computer about 15 minutes to open the data file, but I completed the first run of the key data point in less than five minutes. I was able to drop those findings into the nut graf that I had prepped and immediately felt as though I had a stronger story. As with any other source interview, I had some specific follow-up questions for the data set and spent the rest of the day exploring the findings that showed up.
Having a clear, specific focus kept me grounded in my reporting, calm when it came time for the technical number crunching, and confident enough to continue to chase the data through trials and tribulations. It also helped keep me nimble and efficient in the face of deadlines. While I’m not eager for another public records battle, I do look forward to leveraging the skills I learned through this fellowship — particularly the importance of a focused approach — in many more data-driven stories in the future.