Five key lessons for using data to report on hospital penalty programs
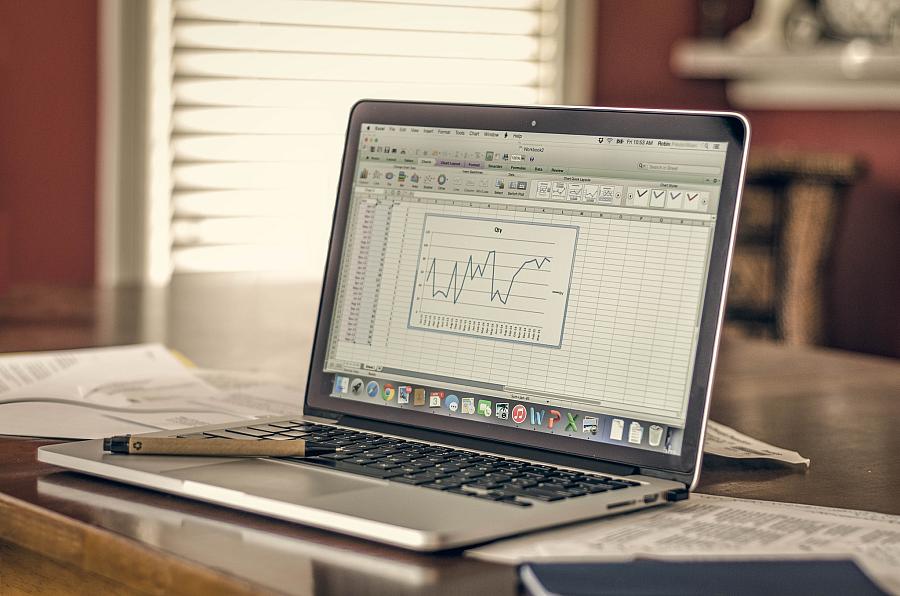
Pay-for-performance programs often sound good in theory. To many people, it seems intuitively reasonable to pay more for a higher quality product and less for a lower quality product — including when that product is health care.
For my fellowship project, I used data to investigate the impact of three federal pay-for-performance programs on hospitals: the Hospital Readmissions Reduction Program, Hospital Value-Based Purchasing, and the Hospital-Acquired Conditions Reduction Program.
Created by the Affordable Care Act, these pay-for-performance programs — also known as penalty programs — aim to improve hospital care on a national level. Hospitals are legally required to report myriad data on patient outcomes, treatment processes, readmissions, and more. Medicare uses this data to compare and score hospitals. And it penalizes those that perform poorly on certain quality metrics by docking their Medicare reimbursements. In 2015, low-scoring hospitals could lose up to 5.5 percent of funds they would otherwise receive from Medicare.
Most hospitals could not afford to stay open without Medicare payments. For hospitals with tight or negative margins, even small losses in revenue can add up to service cuts. That’s why I wanted to explore the disproportionate impact of these penalty programs on two types of hospitals: safety net hospitals, which tend to care for the poorest patients, and major teaching hospitals, which tend to care for the sickest patients.
To understand the impact of these programs on different hospitals, I had to delve into the Medicare data. And although my focus was on these particular Medicare programs, the lessons I learned from this project might apply to many other stories where data plays a prominent role.
1) Get comfortable with the tools and be prepared to troubleshoot.
Every story is an opportunity to learn something new. When I pitched this project, I had some basic knowledge of data analysis. And when I hit snags, I received help from colleagues, other journalists, and the plentiful free resources available on the Internet.
When you are writing a data-driven story on health care or other topics, it’s great to have a working knowledge of tools like Excel. That said, I think you can learn the skills you need using online tutorials and help sites. For example, School of Data offers free tutorials, mainly using Google Spreadsheets. The Center for Health Journalism hosted The Wall Street Journal’s Paul Overberg for a webinar on how to use Excel to analyze health data. The International Consortium of Investigative Journalists also has a great starter guide for basic Excel skills. And if you want in-depth advice on how to work with Medicare’s hospital readmissions data, see Jordan Rau’s explanation in the Columbia Journalism Review.
For the bulk of my project, all I needed was Excel. If you are uncertain about which functions and commands you would need to know for a comparable project, here is a summary of what I used:
- VLOOKUP: This function allows you to combine different datasets that share matching values in a list. For example, Medicare provides data sets for the three penalty programs that contain provider ID numbers, but no hospital names. Medicare also provides a data set called “Hospital General Information” that contains provider ID numbers, with hospital names. Using VLOOKUP, I combined these data sets, so I could see each hospital’s name and the penalties it received in a single spreadsheet. Microsoft Office has a helpful reference card, and many tech blogs offer tips to help people navigate this function. A common VLOOKUP error can occur if cells that appear to contain numbers actually contain text, trailing spaces, or formulas. This is an issue with the Medicare provider ID numbers. This blog offers some quick fixes.
- Functions for basic calculations: I used functions like SUM to find simple information about the penalties, like the approximate total penalties for each hospital. However, some hospitals are in one penalty program, but not the others. I noticed that the SUM function does not automatically count “#N/A” values as zero. You can work around this by using the IF and IS functions.
- IF and IS: For both of these functions, the value of a cell will depend on whether a certain condition is true or false. For example, by using IF and ISNA, you can tell Excel that if a cell contains “#N/A,” it should count the value as zero. Then other functions, like SUM, will work correctly. Here is an example of a formula for that situation.
- Sorting, Filtering, and Conditional Formatting: These tools allow you to rearrange the data and identify trends. As an example, I used conditional formatting to mark all hospitals that have both safety net and major teaching status. Next, I sorted the data based on the size of the combined penalties that these hospitals received. Here’s what the final version of the spreadsheet looked like.
Many investigative stories that use data require more advanced tools than Excel — but some require little more than a calculator. Keep in mind, it can be time-consuming to learn new data management skills or troubleshoot unforeseen issues.
When it comes to seeking additional support, academic researchers are often interested and willing to help. If your analysis reveals a significant trend in the data, it’s a good idea to talk to experts about what you’ve found. They may be open to reviewing your work. For example, a statistics professor ran basic tests on the data analysis for my project, so I could be sure the findings were meaningful. I’m very grateful to the researchers who took the time to offer their help and insight.
2) Putting a face to the data can be a challenge — especially when health care providers are the ones collecting that data.
Even with mountains of data, it’s hard to tell a story without real people who are willing to share their experiences. For my project, one of the biggest challenges was finding sources whose stories were reflected in the data.
Hospitals will sometimes connect reporters with patients for a story, but that wasn’t the case for my project. For one thing, hospitals are bound by HIPAA, which protects individual patients’ health information. Beyond that, I was reporting on penalty programs — and for obvious reasons, few hospitals are interested in highlighting their possible shortcomings or mistakes. Even when I expressed interest in reporting on successful programs that a hospital was running to improve patient care (and quality metrics), administrators declined to put me in touch with patients who had benefitted.
Fortunately, there are other ways to find patient sources. I tried word-of-mouth, contacting patient advocacy groups and nonprofit organizations, and various online forums. However, even when I did find patients whose experiences reflected the data, most were unwilling to share their stories publically — sometimes for practical reasons, like pending medical malpractice litigation.
Ultimately, I had to broaden my criteria and find ways to include personal stories that represented different pieces of the data puzzle. For example, I spoke with a patient who had experienced a life-threatening medical error nine years ago about how it continued to impact her present health. Although it wasn’t the story I had originally planned to tell, her experience still helped put a face to the issue.
3) Understand what the metrics actually measure — but don’t get lost in the weeds.
I spoke with several researchers, hospital administrators, and other journalists who described Medicare’s penalty programs as “simple.” But to me, the programs’ metrics didn’t seem simple at all.
The most complex program, Value-Based Purchasing, has 24 metrics in four different categories. It uses a formula that considers how a hospital performs compared to other hospitals, as well as compared to its own past performance. The hospital’s score is intended to reflect the value and quality of care it provides. (Here is a helpful breakdown if you would like to learn more.).
It seems simpler now — but when I first started looking at the research, I felt lost in the weeds. I knew that I shouldn’t take the metrics at face value and assume they were accurate measures of hospital quality.
This issue is not confined to health care data. In any story that uses data, it’s crucial to understand what is actually being measured. As Jonathan Stray, writing for NeimanLab, points out: metrics “will never exactly represent what is important . . . Metrics are just proxies for our real goals — sometimes quite poor proxies.”
In order to understand why safety net and major teaching hospitals tend to lose in Medicare’s penalty programs (and why small private hospitals tend to win), I needed to understand what each measurement really meant. The bigger questions were: Do these hospitals actually offer worse care? Or are there problems with the metrics? Or does the answer lie somewhere in between?
On the surface, the data suggested that some hospitals might be providing much worse care, with higher rates of certain medical errors and inferior scores on patient satisfaction surveys. But as I dug deeper into research on the metrics, my perspective changed.
For example, several of the metrics for medical errors may be prone to “surveillance bias,” including those that count bed sores and blood clots following surgery. That means the more hospitals try to identify and address those medical errors, the more errors they find and report to Medicare, and the worse they score. Conversely, hospitals that don’t actively work to identify errors could potentially score better in those areas.
When I looked into patient satisfaction surveys, the issues were even clearer. Understandably, patients who have private rooms and gourmet food options give their hospitals’ higher ratings, compared to patients sleeping in four-bed wards. However, such perks don’t always translate into better health outcomes.
I’m glad I stayed in the weeds long enough to gain perspective on these issues. However, I may have lingered too long and lost valuable time. If you’re working with data, it’s vital to understand the metrics used to collect it. On the other hand, stay aware of your deadline and avoid getting caught up in minutiae.
4) If the data doesn’t exist, it may be necessary to change course.
One of my greatest regrets for this project is that I wasn’t able to address the issue of uncompensated care, or charity care, in the series. Uncompensated care refers to medical care for which hospitals do not receive payment — often because patients are uninsured.
Major teaching and safety net hospitals tend to provide a disproportionate amount of this type of care, compared to other hospitals. In some regions, these hospitals are the only facilities where uninsured people can get the medical help they need.
I originally planned to write at least one story focused on uncompensated care. Unfortunately, there is no national system in place to track rates of uncompensated care at hospitals, and it wasn’t practical to collect uncompensated care statistics from individual institutions. Although I worked toward finding other ways to include this issue in my project, I ultimately had to let it go.
It was difficult to set aside an angle in which I had invested significant time and energy. But I needed to focus on areas of my project where I actually had data and insights to share.
5) Keep moving forward
The issues I tried to tackle in this project were complex, and I often focused too much on small details. I was so interested in the data that I may have tried to cover too many different aspects of it in my project. As Sandra Crucianelli notes in a great introductory guide to data journalism, “Sometimes it's not just about presenting data, but letting your audience see what it means to them.”
In hindsight, I wish I had been more flexible, rather than laboring over story ideas that didn’t work out. At the same time, even though I encountered obstacles, I kept moving forward and looking for new ways to tell the story. Ultimately, my goal was to report on complicated public policies in a way that made the issues more accessible and relatable. Although my project did not come together exactly as I planned, I was glad to provide another perspective on these issues.
[Creative Commons photo by Grant via Flickr.]