Tracking COVID-19 models can feel like a brutal roller coaster. Is that their fault?
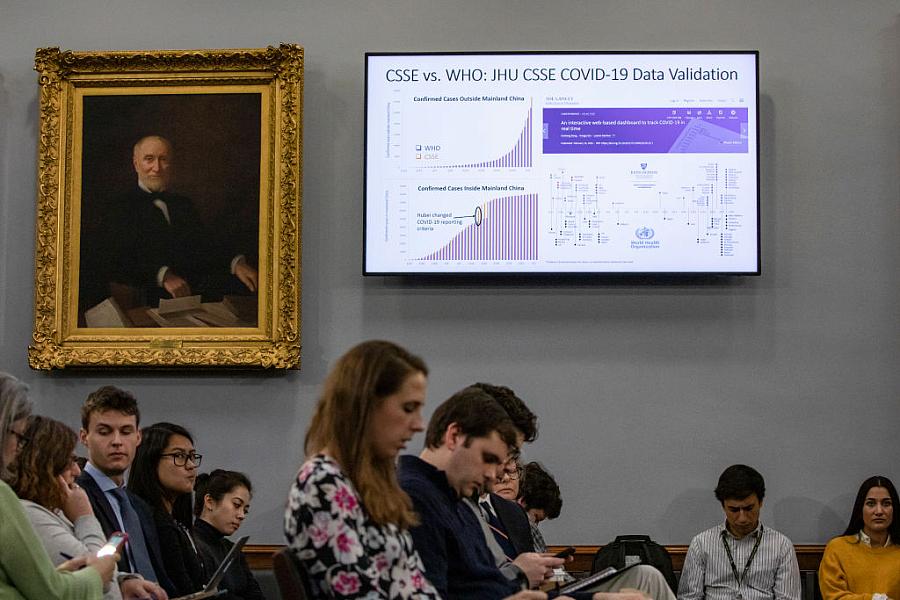
Researchers gather at a COVID-19 briefing at Johns Hopkins University on March 6, when there were only 14 reported U.S. deaths. Predictions of what came next were wildly divergent and constantly revised.
(Photo by Samuel Corum/Getty Images)
As the pandemic wears on, we are all hungry for information about what the future is going to look like. Will we see another big wave of infections? How careful must we be and when might this end? Sophisticated models can help us start to break down these questions, but the flip side is that no model can answer them with certainty.
In recent weeks, frustration has grown as models from diverse sources don’t match up and sometimes dramatically change over time. In particular, one influential model frequently cited by the White House appeared to swing wildly from one estimate to another. Created by the University of Washington’s Institute for Health Metrics and Evaluation (IMHE), the model has been most repeatedly criticized for underestimating the pandemic’s severity and changing its predictions.
In late April, the model predicted the pandemic would have a cumulative national death toll of only about 60,000 (the model’s uncertainty estimates included a range of anywhere between about 30,000 and 126,000), with deaths dropping to zero in some places by late spring. Only a few days later, on May 4, that number of projected deaths had risen to 135,000. At the time of this writing the official U.S. death toll, known to be an undercount, is nearing 100,000 and rising.
Some critics of the IMHE model say its optimism emboldened leaders eager to reopen states and drop stay-at-home orders much sooner than was safe. As of this writing, the model now estimates we’ll see more than 131,967 deaths by August 4.
In a written statement published alongside their May 4 update, the IHME team attributed the dramatic changes in its numbers in part to more and better data on COVID-19 deaths, hospitalizations and cases, and data on “human mobility patterns.” They also acknowledge the pandemic has played out very differently in different parts of the world, a problem for their original approach, which used outbreak trajectories from early-hit nations to predict what the U.S. outbreak might look like.
“There’s a ton of stuff that nobody understands about this pandemic,” said IHME director Chris Murray, who added that it shouldn’t be surprising to see the numbers move around as our understanding of the disease advances. “We’re adapting as the data comes in and as we hear various scientific critiques.”
That’s not unusual in modeling outbreaks. “There’s totally no shame in changing and updating a model over time,” said Daniela Witten, a biostatistician at the University of Washington School of Public Health in Seattle who is unaffiliated with IMHE. But she sees the IHME story as a cautionary tale of what can happen when relying too heavily on one model alone. All models rely on a set of imperfect assumptions to arrive at their conclusions. Picking one model to follow at the exclusion of others shows a lack of understanding of the limitations inherent to any model, she said. That approach can be particularly misleading when the model selected is an outlier with rosier projections than the rest.
“It’s disheartening and alarming when we see politicians seizing upon one particular model at one snapshot in time,” Witten says. “They should not be cherry-picking just the one model that agrees with their political viewpoint.”
Instead, leaders should recognize that all models have strengths and weaknesses and carefully consider the range of models at their disposal, said Erin Mordecai, an infectious disease ecologist at the Stanford Woods Institute for the Environment, whose lab has applied their own COVID-19 model to Santa Clara County.
Different kinds of models are good at doing different things, she says. So-called SIR or SEIR models consider the amount of people likely to be susceptible (S), infected (I) or recovered or deceased (R) at any given time (the SEIR variation adds “E” for exposed). Such models estimate outbreak trajectories by looking at what is known about how a disease spreads and how human behavior affects that spread. Another approach, called agent-based modeling, simulates human behavior in a more detailed way and models how people might move through the world and interact with each other.
“It’s disheartening and alarming when we see politicians seizing upon one particular model at one snapshot in time. They should not be cherry-picking just the one model that agrees with their political viewpoint.” — Daniela Witten, the University of Washington School of Public Health
The IHME model began as a statistical, curve-fitting model, which means researchers based their predictions on the outbreak trajectory seen in early-hit nations. Such a model can be good for making short-term predictions about hospital needs, says Mordecai, but because it doesn’t incorporate how disease transmission works, it lacks flexibility and can’t respond as well to potential shifts in behaviors, like stay-at-home or orders or mandating masks. The IHME’s model now mixes their original, statistical approach with a new SEIR component, which incorporates more information about disease transmission.
One problem with the IMHE’s early “curve fitting” approach, which forces data to fit a certain, predetermined trajectory shape, is that it led to models that assumed an outbreak with a single crest. These kinds of depictions lead us to believe that we’ll see a “peak” in deaths or cases, with the assumption that after the peak, new cases and deaths will decline steadily towards zero — in April, IMHE projected zero deaths in California by May 20, which clearly didn’t happen. That modeling approach fails to account for what will happen as lockdowns and social distancing measures are lifted, Mordecai said.
“I think there’s this misconception that once we're past the peak, we can just stop everything, but I think if we were to do that we would just head towards a second, much bigger peak,” she says. “If you were to think about how many people would get infected in the population if we did nothing to control the epidemic, that’s kind of the ultimate peak. We're nowhere near that yet, and we don't want to be because that would mean millions of people dying.”
Ultimately, we’d hope that our epidemiological models would give us predictions akin to weather forecasts, but accounting for changes in human behavior complicates estimates. Staying home or bringing an umbrella won’t change the likelihood of a bad storm hitting, but staying home or wearing a mask can change the course of an epidemic.
Weather forecasting also typically takes into account many models and shared data, an approach disease forecasting would ideally take as well said Thomas McAndrew, a postdoctoral biostatistician at the University of Massachusetts Amherst. He’s part of a team trying to push in this direction by pulling together results of prominent COVID models to output one “ensemble” model. They’re also using surveys to aggregate weekly assessments from infectious disease experts across the United States.
“My personal opinion is that ensemble modeling is the way to go,” said McAndrew, who compared it to buying a house. “I don’t want to be looking at just one website to find the best neighborhood to live in. People collect many opinions and different assumptions and ideas from all sorts of people. The same goes for this too.”
For more information on how to cover COVID-19 models, check out this tip sheet from Journalist’s Resource.