In scramble to respond to Covid-19, hospitals turned to models with high risk of bias
This story is part of a larger project led by Elise Reuter, a 2020 Data Fellow who is investigating what clinical-decision support tools hospitals are using for COVID-19 patients, how they are implemented, and whether they are evaluated for potential bias.
Her other stories include:
Part 2: FDA faces big questions as it takes a closer look at AI regulations
Part 3: Hit hard by the pandemic, one California county looks to vaccinate its most vulnerable
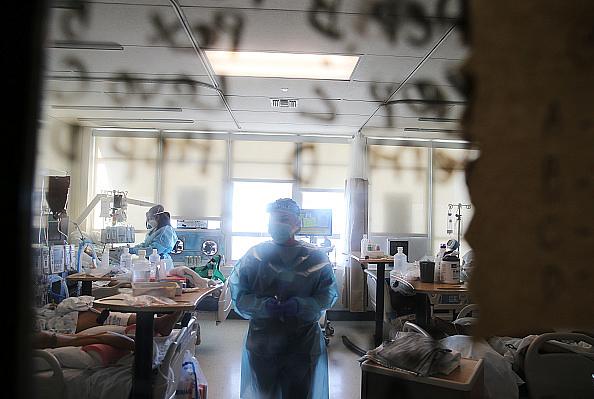
Clinicians care for Covid-19 patients in a makeshift ICU at Harbor-UCLA Medical Center on January 21, 2021 in Torrance, California. As cases surged in the winter months, some hospitals turned to models to help predict which patients faced an increased risk of becoming critically ill.
Mario Tama, Getty Images
A year ago, Michigan Medicine faced a dire situation. In March of 2020, the health system predicted it would have three times as many patients as its 1,000-bed capacity — and that was the best-case scenario. Hospital leadership prepared for this grim prediction by opening a field hospital in a nearby indoor track facility, where patients could go if they were stable, but still needed hospital care. But they faced another predicament: How would they decide who to send there?
Two weeks before the field hospital was set to open, Michigan Medicine decided to use a risk model developed by Epic Systems to flag patients at risk of deterioration. Patients were given a score of 0 to 100, intended to help care teams determine if they might need an ICU bed in the near future. Although the model wasn’t developed specifically for Covid-19 patients, it was the best option available at the time, said Dr. Karandeep Singh, an assistant professor of learning health sciences at the University of Michigan and chair of Michigan Medicine’s clinical intelligence committee. But there was no peer-reviewed research to show how well it actually worked.
Researchers tested it on over 300 Covid-19 patients between March and May. They were looking for scores that would indicate when patients would need to go to the ICU, and if there was a point where patients almost certainly wouldn’t need intensive care.
“We did find a threshold where if you remained below that threshold, 90% of patients wouldn’t need to go to the ICU,” Singh said. “Is that enough to make a decision on? We didn’t think so.”
But if the number of patients were to far exceed the health system’s capacity, it would be helpful to have some way to assist with those decisions.
“It was something that we definitely thought about implementing if that day were to come,” he said in a February interview.
Thankfully, that day never came.
The survey
Michigan Medicine is one of 80 hospitals contacted by MedCity News between January and April in a survey of decision-support systems implemented during the pandemic. Of the 26 respondents, 12 used machine learning tools or automated decision systems as part of their pandemic response. Larger hospitals and academic medical centers used them more frequently.
Faced with scarcities in testing, masks, hospital beds and vaccines, several of the hospitals turned to models as they prepared for difficult decisions. The deterioration index created by Epic was one of the most widely implemented — more than 100 hospitals are currently using it — but in many cases, hospitals also formulated their own algorithms.
They built models to predict which patients were most likely to test positive when shortages of swabs and reagents backlogged tests early in the pandemic. Others developed risk-scoring tools to help determine who should be contacted first for monoclonal antibody treatment, or which Covid patients should be enrolled in at-home monitoring programs.
MedCity News also interviewed hospitals on their processes for evaluating software tools to ensure they are accurate and unbiased. Currently, the FDA does not require some clinical decision-support systems to be cleared as medical devices, leaving the developers of these tools and the hospitals that implement them responsible for vetting them.
Among the hospitals that published efficacy data, some of the models were only evaluated through retrospective studies. This can pose a challenge in figuring out how clinicians actually use them in practice, and how well they work in real time. And while some of the hospitals tested whether the models were accurate across different groups of patients — such as people of a certain race, gender or location — this practice wasn’t universal.
//
As more companies spin up these models, researchers cautioned that they need to be designed and implemented carefully, to ensure they don’t yield biased results.
An ongoing review of more than 200 Covid-19 risk-prediction models found that the majority had a high risk of bias, meaning the data they were trained on might not represent the real world.
“It’s that very careful and non-trivial process of defining exactly what we want the algorithm to be doing,” said Ziad Obermeyer, an associate professor of health policy and management at UC Berkeley who studies machine learning in healthcare. “I think an optimistic view is that the pandemic functions as a wakeup call for us to be a lot more careful in all of the ways we’ve talked about with how we build algorithms, how we evaluate them, and what we want them to do.”
Algorithms can’t be a proxy for tough decisions
Concerns about bias are not new to healthcare. In a paper published two years ago, Obermeyer found a tool used by several hospitals to prioritize high-risk patients for additional care resources was biased against Black patients. By equating patients’ health needs with the cost of care, the developers built an algorithm that yielded discriminatory results.
More recently, a rule-based system developed by Stanford Medicine to determine who would get the Covid-19 vaccine first ended up prioritizing administrators and doctors who were seeing patients remotely, leaving out most of its 1,300 residents who had been working on the front lines. After an uproar, the university attributed the errors to a “complex algorithm,” though there was no machine learning involved.
Both examples highlight the importance of thinking through what exactly a model is designed to do — and not using them as a proxy to avoid the hard questions.
“The Stanford thing was another example of, we wanted the algorithm to do A, but we told it to do B. I think many health systems are doing something similar,” Obermeyer said. “You want to give the vaccine first to people who need it the most — how do we measure that?”
The urgency that the pandemic created was a complicating factor. With little information and few proven systems to work with in the beginning, health systems began throwing ideas at the wall to see what works. One expert questioned whether people might be abdicating some responsibility to these tools.
“Hard decisions are being made at hospitals all the time, especially in this space, but I’m worried about algorithms being the idea of where the responsibility gets shifted,” said Varoon Mathur, a technology fellow at NYU’s AI Now Institute, in a Zoom interview. “Tough decisions are going to be made, I don’t think there are any doubts about that. But what are those tough decisions? We don’t actually name what constraints we’re hitting up against.”
The wild, wild west
There currently is no gold standard for how hospitals should implement machine learning tools, and little regulatory oversight for models designed to support physicians’ decisions, resulting in an environment that Mathur described as the “wild, wild west.”
How these systems were used varied significantly from hospital to hospital.
Early in the pandemic, Cleveland Clinic used a model to predict which patients were most likely to test positive for the virus as tests were limited. Researchers developed it using health record data from more than 11,000 patients in Ohio and Florida, including 818 who tested positive for Covid-19. Later, they created a similar risk calculator to determine which patients were most likely to be hospitalized for Covid-19, which was used to prioritize which patients would be contacted daily as part of an at-home monitoring program.
Initially, anyone who tested positive for Covid-19 could enroll in this program, but as cases began to tick up, “you could see how quickly the nurses and care managers who were running this program were overwhelmed,” said Dr. Lara Jehi, Chief Research Information Officer at Cleveland Clinic. “When you had thousands of patients who tested positive, how could you contact all of them?”
While the tool included dozens of factors, such as a patient’s age, sex, BMI, zip code, and whether they smoked or got their flu shot, it’s also worth noting that demographic information significantly changed the results. For example, a patient’s race “far outweighs” any medical comorbidity when used by the tool to estimate hospitalization risk, according to a paper published in Plos One. Cleveland Clinic recently made the model available to other health systems.
Others, like Stanford Health Care and 731-bed Santa Clara County Medical Center, started using Epic’s clinical deterioration index before developing their own Covid-specific risk models. At one point, Stanford developed its own risk-scoring tool, which was built using past data from other patients who had similar respiratory diseases, such as the flu, pneumonia, or acute respiratory distress syndrome. It was designed to predict which patients would need ventilation within two days, and someone’s risk of dying from the disease at the time of admission.
Stanford tested the model to see how it worked on retrospective data from 159 patients that were hospitalized with Covid-19, and cross-validated it with Salt Lake City-based Intermountain Healthcare, a process that took several months. Although this gave some additional assurance — Salt Lake City and Palo Alto have very different populations, smoking rates and demographics — it still wasn’t representative of some patient groups across the U.S.
“Ideally, what we would want to do is run the model specifically on different populations, like on African Americans or Hispanics and see how it performs to ensure it’s performing the same for different groups,” Tina Hernandez-Boussard, an associate professor of medicine, biomedical data science and surgery at Stanford, said in a February interview. “That’s something we’re actively seeking. Our numbers are still a little low to do that right now.”
Stanford planned to implement the model earlier this year, but ultimately tabled it as Covid-19 cases fell.
‘The target is moving so rapidly’
Although large medical centers were more likely to have implemented automated systems, there were a few notable holdouts. For example, UC San Francisco Health, Duke Health and Dignity Health all said they opted not to use risk-prediction models or other machine learning tools in their pandemic responses.
“It’s pretty wild out there and I’ll be honest with you — the dynamics are changing so rapidly,” said Dr. Erich Huang, chief officer for data quality at Duke Health and director of Duke Forge. “You might have a model that makes sense for the conditions of last month but do they make sense for the conditions of next month?”
That’s especially true as new variants spread across the U.S., and more adults are vaccinated, changing the nature and pace of the disease. But other, less obvious factors might also affect the data. For instance, Huang pointed to big differences in social mobility across the state of North Carolina, and whether people complied with local restrictions. Differing social and demographic factors across communities, such as where people work and whether they have health insurance, can also affect how a model performs.
“There are so many different axes of variability, I’d feel hard pressed to be comfortable using machine learning or AI at this point in time,” he said. “We need to be careful and understand the stakes of what we’re doing, especially in healthcare.”
Leadership at one of the largest public hospitals in the U.S., 600-bed LAC+USC Medical Center in Los Angeles, also steered away from using predictive models, even as it faced an alarming surge in cases over the winter months.
At most, the hospital used alerts to remind physicians to wear protective equipment when a patient has tested positive for Covid-19.
“My impression is that the industry is not anywhere near ready to deploy fully automated stuff just because of the risks involved,” said Dr. Phillip Gruber, LAC+USC’s chief medical information officer. “Our institution and a lot of institutions in our region are still focused on core competencies. We have to be good stewards of taxpayer dollars.”
When the data itself is biased
Developers have to contend with the fact that any model developed in healthcare will be biased, because the data itself is biased; how people access and interact with health systems in the U.S. is fundamentally unequal.
How that information is recorded in electronic health record systems (EHR) can also be a source of bias, NYU’s Mathur said. People don’t always self-report their race or ethnicity in a way that fits neatly within the parameters of an EHR. Not everyone trusts health systems, and many people struggle to even access care in the first place.
“Demographic variables are not going to be sharply nuanced. Even if they are… in my opinion, they’re not clean enough or good enough to be nuanced into a model,” Mathur said.
The information hospitals have had to work with during the pandemic is particularly messy. Differences in testing access and missing demographic data also affect how resources are distributed and other responses to the pandemic.
“It’s very striking because everything we know about the pandemic is viewed through the lens of number of cases or number of deaths,” UC Berkeley’s Obermeyer said. “But all of that depends on access to testing.”
At the hospital level, internal data wouldn’t be enough to truly follow whether an algorithm to predict adverse events from Covid-19 was actually working. Developers would have to look at social security data on mortality, or whether the patient went to another hospital, to track down what happened.
“What about the people a physician sends home — if they die and don’t come back?” he said.
Researchers at Mount Sinai Health System tested a machine learning tool to predict critical events in Covid-19 patients — such as dialysis, intubation or ICU admission — to ensure it worked across different patient demographics. But they still ran into their own limitations, even though the New York-based hospital system serves a diverse group of patients.
They tested how the model performed across Mount Sinai’s different hospitals. In some cases, when the model wasn’t very robust, it yielded different results, said Benjamin Glicksberg, an assistant professor of genetics and genomic sciences at Mount Sinai and a member of its Hasso Plattner Institute for Digital Health.
They also tested how it worked in different subgroups of patients to ensure it didn’t perform disproportionately better for patients from one demographic.
“If there’s a bias in the data going in, there’s almost certainly going to be a bias in the data coming out of it,” he said in a Zoom interview. “Unfortunately, I think it’s going to be a matter of having more information that can approximate these external factors that may drive these discrepancies. A lot of that is social determinants of health, which are not captured well in the EHR. That’s going to be critical for how we assess model fairness.”
Even after checking for whether a model yields fair and accurate results, the work isn’t done yet. Hospitals must continue to validate continuously to ensure they’re still working as intended — especially in a situation as fast-moving as a pandemic.
A bigger role for regulators
All of this is stirring up a broader discussion about how much of a role regulators should have in how decision-support systems are implemented.
Currently, the FDA does not require most software that provides diagnosis or treatment recommendations to clinicians to be regulated as a medical device. Even software tools that have been cleared by the agency lack critical information on how they perform across different patient demographics.
Of the hospitals surveyed by MedCity News, none of the models they developed had been cleared by the FDA, and most of the external tools they implemented also hadn’t gone through any regulatory review.
In January, the FDA shared an action plan for regulating AI as a medical device. Although most of the concrete plans were around how to regulate algorithms that adapt over time, the agency also indicated it was thinking about best practices, transparency, and methods to evaluate algorithms for bias and robustness.
More recently, the Federal Trade Commission warned that it could crack down on AI bias, citing a paper that AI could worsen existing healthcare disparities if bias is not addressed.
“My experience suggests that most models are put into practice with very little evidence of their effects on outcomes because they are presumed to work, or at least to be more efficient than other decision-making processes,” Kellie Owens, a researcher for Data & Society, a nonprofit that studies the social implications of technology, wrote in an email. “I think we still need to develop better ways to conduct algorithmic risk assessments in medicine. I’d like to see the FDA take a much larger role in regulating AI and machine learning models before their implementation.”
Developers should also ask themselves if the communities they’re serving have a say in how the system is built, or whether it is needed in the first place. The majority of hospitals surveyed did not share with patients if a model was used in their care or involve patients in the development process.
In some cases, the best option might be the simplest one: don’t build.
In the meantime, hospitals are left to sift through existing published data, preprints and vendor promises to decide on the best option. To date, Michigan Medicine’s paper is still the only one that has been published on Epic’s Deterioration Index.
Care teams there used Epic’s score as a support tool for its rapid response teams to check in on patients. But the health system was also looking at other options.
“The short game was that we had to go with the score we had,” Singh said. “The longer game was, Epic’s deterioration index is proprietary. That raises questions about what is in it.”
Elise Reuter reported this story while participating in the USC Annenberg Center for Health Journalism’s 2020 Data Fellowship.
The Center for Health Journalism supports journalists as they investigate health challenges and solutions in their communities. Our online community hosts an interdisciplinary conversation about community health, social determinants, child and family well-being and journalism.
[This story was originally published by MedCity News.]
Did you like this story? Your support means a lot! Your tax-deductible donation will advance our mission of supporting journalism as a catalyst for change.