The algorithms used to calculate Covid-19 risk might be prone to bias
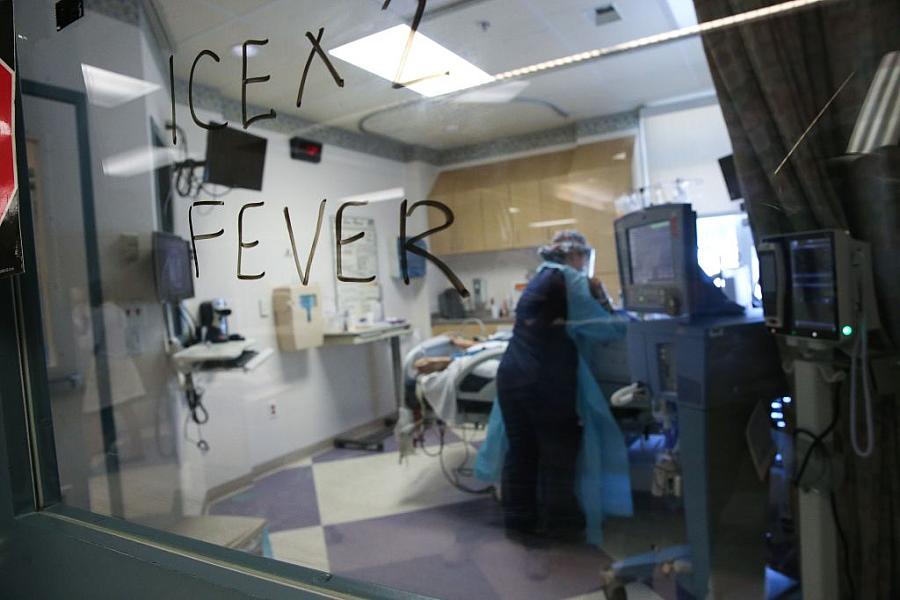
A nurse cares for a COVID-19 patient at El Centro Regional Medical Center in hard-hit Imperial County in July in El Centro, California. The rural county, which is 85 percent Latino, borders Mexico and Arizona and endures high poverty rates and air pollution while also being medically underserved.
(Photo by Mario Tama/Getty Images)
Hospitals and insurance companies use a variety of algorithms to calculate risk, but they don’t always yield equitable results. Last year, researchers discovered an algorithm developed by Optum and used by several hospitals was racially biased.
The algorithm, intended to identify patients who would benefit from extra care based on how much they might cost the health system in the future, may have been biased because health spending on Black patients was lower than spending on white patients. The researchers’ findings sparked a chain of investigations, but little has been shared since. It’s not clear whether these algorithms are still in use.
Now, hospitals are adopting a new set of models to help manage growing numbers of COVID-19 patients. Several tools were quickly developed during the pandemic to triage patients or assess their risk of becoming critically ill. For example, the Cleveland Clinic recently released a model it developed to predict whether a patient was likely to test positive for COVID-19.
Another tool created by electronic health record systems giant Epic is already being used by at least 70 hospitals. It’s intended to help predict which COVID-19 patients should be transferred to the ICU based on their vitals, but some hospitals have built on this tool to also predict which patients can be discharged early.
The developers behind these models said they were careful to pull data from several states and backgrounds to ensure they would be broadly applicable. But it is not clear whether health systems test these tools to ensure they don’t discriminate against some patients, and they’re not always implemented in the same way.
The vast majority of these tools have not been cleared — let alone reviewed — by the Food and Drug Administration.
Earlier this year, a group of researchers reviewed 145 COVID-19 prediction models. Based on their findings, they did not recommend using them.
“This review indicates that proposed models are poorly reported, at high risk of bias, and their reported performance is probably optimistic,” they wrote in their review, published in the BMJ.
There are a multitude of reasons why health care algorithms might yield biased results. Existing inequities, such as underserved groups receiving fewer resources, can easily filter into predictive tools, such as in the case of Optum’s algorithm. Data pulled from electronic health record systems might also have gaps, particularly for underserved patients.
Underrepresentation is also a concern, both in the data itself and the people who are developing AI tools. If patients aren’t able to access testing or health care resources, their experiences might not be reflected in the data.
For my 2020 Data Fellowship project, I plan to identify testing gaps in Southern California by identifying communities with low per-capita testing rates and pairing that with data on insurance coverage and access to transportation. This information can help provide a baseline for who is being underrepresented in testing data, which has been the foundation for policy decisions and many triage tools developed for hospitals.
Imperial County, which spans the mountains and dunes between San Diego and Phoenix, was identified as a “testing desert” by the state in March. Since then, it has opened an additional testing site. It currently has a very high test positivity rate, at 16.5%.
Just north of that is Riverside County, whose public health department sent out a memo last month that more residents needed to be tested after it fell short of California’s average testing volume.
I will also survey hospitals on what clinical-decision support tools they are using for COVID-19 patients, how they are implemented, and whether they are evaluated for potential bias. For hospitals that have tested the efficacy of these tools, I will also request that data. A few have shared preprints on how successful these tools were for predicting patient outcomes.
The use of these systems, whether in a pandemic or another context, also brings up some thorny questions. For example, is it the responsibility of the developer or the hospital using the algorithm to ensure it is not biased? Should patients be notified that one of these tools is used as part of their care?
The good news is that there are ways to test algorithms to ensure they don’t yield discriminatory results. For example, Rayid Ghani, a professor at Carnegie Mellon University’s Machine Learning Department, advises clearly defining the goals of an AI system upfront and auditing them to make sure that was what the tool actually accomplished.
I hope this work will result in greater transparency in how health care algorithms are developed and implemented, and give underrepresented patients a greater role in determining how they are used.